AI-native technology is shaking up the debt resolution industry. But what exactly is it and how does it differ from embedded systems? Read on to discover the fundamentals of this pioneering technology.
Key takeaways:
- AI-native systems are built with AI across every element of their architecture.
- This differs from embedded AI, which retrofits AI components into existing tech.
- AI-native systems can continuously learn in real-time, allowing them to be adaptive and dynamic.
- At Ophelos, we’ve pioneered the industry's first AI-native platform.
- Our tech enables a high degree of personalisation at scale, improving customer experience and business outcomes.
Over the last 5 years, AI-driven systems have revolutionised the debt collection landscape.
By automating once resource-intensive processes, AI has enabled collectors to enhance the customer experience and optimise collection journeys — from initial outreach to client-facing analytics.
However, to date, AI within debt resolution has relied on embedded AI systems, with AI only being utilised for certain aspects of their functionality.
At Ophelos, we’re pioneering a new model — an AI-native platform.
In this article, we’ll explain what this relatively new term means, how it fundamentally differs from embedded AI systems, explore its architecture and what makes it superior, as well as touch on how this tech is transforming the industry.
What is AI-native tech?
‘AI-native’ is a term used to describe technology systems that have AI built into every aspect of their architecture.
Unlike legacy AI or embedded AI technologies that integrate AI as added-on components (often as upgrades to existing systems), AI-native technologies are built with artificial intelligence at their core.
With AI being not just a feature, but the foundation for the entire system, AI-native platforms are designed to handle complex, end-to-end data-driven decision-making that utilises AI across multiple system functions.
Sitting right at the frontier of AI’s capabilities, these systems continuously employ machine learning (ML) and reasoning to optimise and improve processes. This means the system can learn and adapt as it runs, without needing specific instructions to do so.
For example, facial recognition is a form of machine learning. Once an algorithm has paired up your details with a photograph of you, it will be able to automatically tag photos of you, without explicitly needing your instructions to do so.
AI-native vs embedded AI
AI-native systems are vastly different to platforms that utilise embedded AI, which is also referred to as AI-driven or AI-enhanced.
Embedded systems retrofit AI into existing operations. This could include replacing current workflows with an AI component or adding an entirely new AI-driven component into an existing technology stack.
Whilst embedded AI can improve performance and functionality, via automation, analytics or improved data outputs — embedded systems tend to be more limited in scope, with AI restricted to specific predefined tasks.
AI-native systems build their entire architecture around AI, with AI present in every component of the system from the start. Whilst embedded systems aim to utilise AI to improve the preexisting platform, AI-native technologies aim to transform the approach entirely.
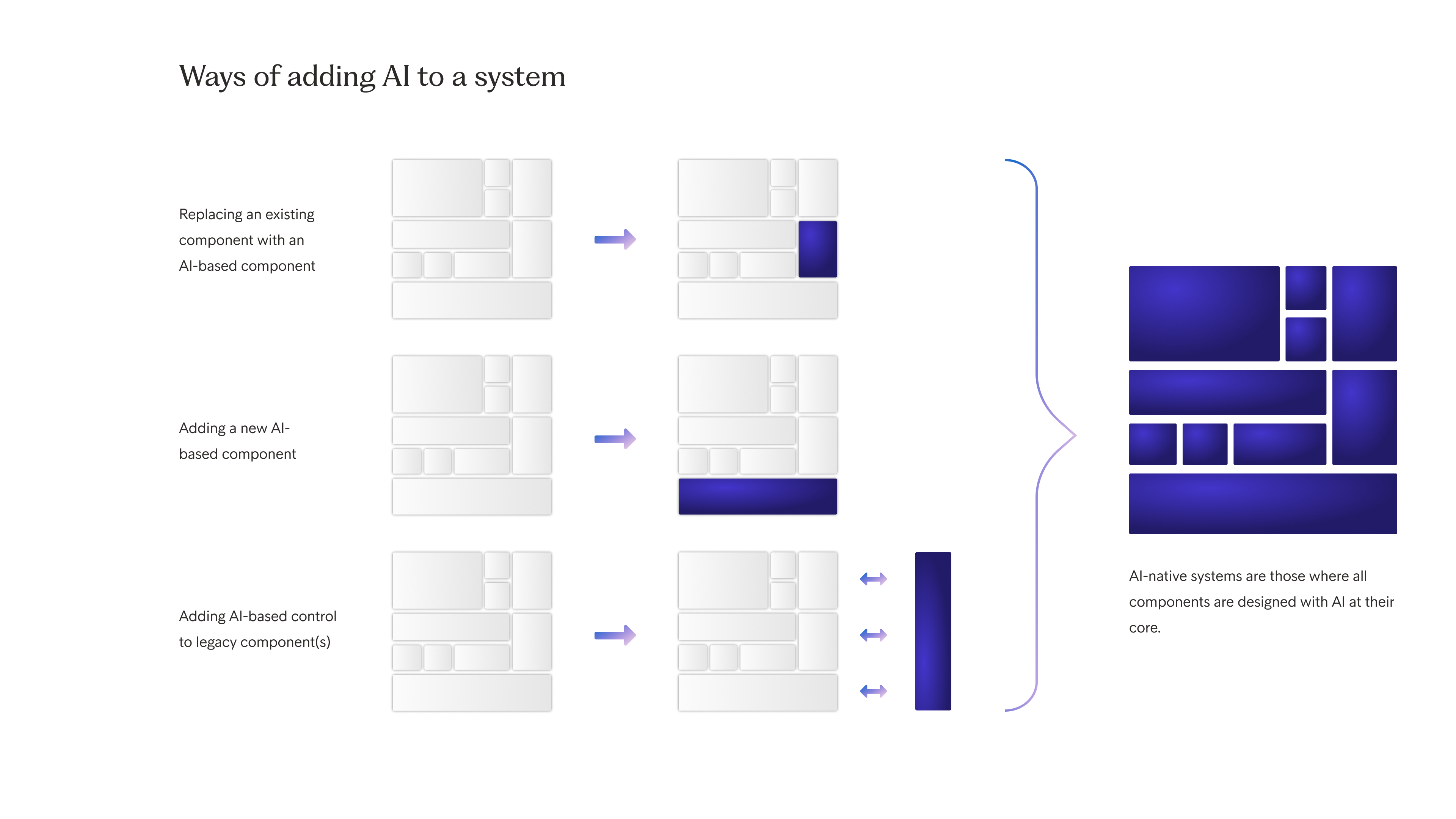
AI-native architecture
AI-native platforms have several distinct architectural features that make them superior to embedded systems.
This includes:
- Distributed data infrastructure
- Knowledge-based ecosystem
- Zero-touch
- Hyper automation intelligence & AIOps
Essentially, AI-native systems are designed to have fully autonomous network infrastructure and employ AI continuously, in real-time, end-to-end.
This allows AI-native systems to be adaptive and dynamic, responding and optimising in response to the input of new data and environment conditions.
What are the benefits of AI-native systems?
Scalability
AI-native platforms, by design, are inherently future-proofed. By reducing human intervention and relying on a far greater degree of automation, native systems can scale faster and more efficiently.
Dynamic responses
Rather than relying on predetermined parameters, AI-native systems are built to be dynamic. By employing continuous optimisation, they adjust systems and strategies in real-time, often in response to customer behaviours. This allows for a higher degree of personalisation and highly targeted actions.
Continuous machine learning
AI-native platforms utilise and learn continuously from results and feedback data. They analyse every customer interaction and can adjust and optimise autonomously. This reduces the need for human input whilst continuously improving performance across a range of tasks.
AI-native systems in debt resolution
The debt resolution industry has been slow to adopt AI, with only a handful of companies integrating AI elements into their digital journeys.
While these components can provide enhanced automation and improved performance via initiating things like automated communications, payment reminders or even generative AI responses for human agents to send out, AI-driven platforms can only go so far.
They are still heavily reliant on large customer service teams and human agents in order to define their parameters and optimise component elements.
However, AI-native platforms, like Ophelos, can employ machine learning and reasoning to autonomously optimise and dynamically respond to customer interactions.
This approach allows for a highly personalised approach to be deployed simultaneously across an entire customer base or journey — improving both customer experience and client outcomes.
Optimising with Olive 2.0
For example, Olive 2.0, our proprietary Large Language Model (LLM) and industry first, can scan customer interactions and proactively predict a customer's vulnerability risk profile.
Olive 2.0 flags vulnerabilities in real-time, flagging to human agents those that need additional support. By summarising interaction histories and providing next-action recommendations for each customer, Olive 2.0 automates several time-intensive processes while ensuring each customer gets the correct level of support.
Olive 2.0 will continue to update a customer’s profile in real-time whenever they engage, allowing their risk profile to always be up-to-date.
Automating at scale
Taking into consideration Olive 2.0’s recommendations, our communications decision engine will then calculate the best time, channel and communication method for contacting each customer.
Depending on their vulnerability risk, customers will either be triaged to customer service agents for additional support or contacted using our generative AI GPT model.
Real-time analysis
Updating performance metrics in real-time, our analytics dashboard allows clients to track collections and engagements as they happen.
Drawing on data from both Olive and our GPT model, clients can easily visualise both macro and micro trends across every customer interaction.
The future is AI-native
Whilst embedded AI has enabled the debt industry and many other financial services to enhance performance via automation and improved efficiency, the future lies with dynamic, end-to-end native systems.
With the power to deliver granular personalisation at scale and continuously optimise with little need for human intervention, AI-native platforms are driving a new frontier for debt resolution.